Inquire
How can improve data quality
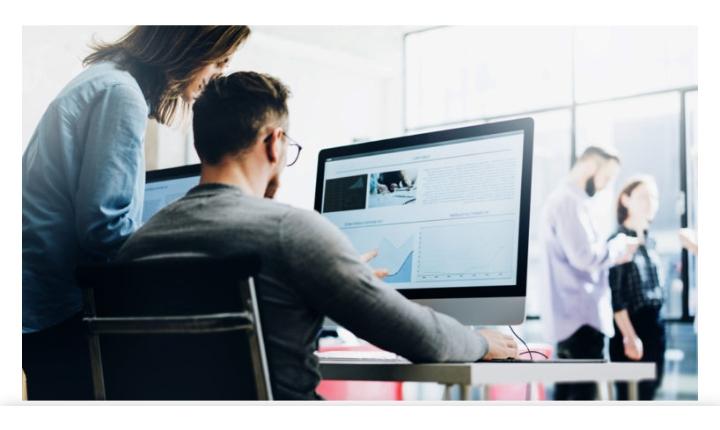
Understanding data quality: key concepts and metrics
The concept of data quality has evolved since its invention in the days of early data processing when quality control was often based on manual inspection. The rise of data, and especially AI-powered applications, has led to an increased focus on data quality as organizations seek to derive value from large data sets.
what is a ata quality strategy
Data quality strategy defines systems and processes to incorporate data quality into all organizational activities to ensure the use of trusted data across the enterprise. An effective data quality strategy captures business goals, objectives, initiatives, activities, roles, and scope to improve data quality and integrity. Defining a data quality strategy helps identify, resolve, and prevent quality issues for building the foundation of trusted data. Below are our top tips for improving data quality to get the best out of your data investments.
Step 1: Define business needs and assess the impact of data quality
Business needs are often the drivers for data quality improvement initiatives. You can prioritize data quality issues according to your business needs and how they will impact your business in the long run. Measuring business impact helps establish a goal and track the progress of data quality improvement. A continued reference to the business needs sets the context for refining the approach to data quality.
Step 2: Develop a comprehensive data quality strategy
For trusted use, you not only need data that is “right” but you also need the “right” data. Yes, not all data is equal. You need to understand data correctly to see if it is “right” or relevant for your intended use. The key here is in understanding your data. Where it comes from, what it describes, and how you can extract the most value from it. is the ability to understand and use your data in the right way. Correctly describing and connecting data throughout its journey is the best strategic approach to improve data quality.
Step 3: Address data quality at the source
Very often, data quality issues get fixed temporarily, only to move on with the work. Consider what happens if a data scientist finds empty records in a selected data set. Most likely, she’ll fix the error in her copy and continue with the analysis. If the corrections do not reach the source, the original data set still retains the quality issue, affecting its subsequent use. Prevention is better than cure, and preventing the propagation of bad data is how you can improve data quality in such cases. Let’s take another case where a health clinic staff often had difficulties contacting the patients after their visits. When they found the phone numbers were wrong for several patients, they decided to address this issue at the root. When patients checked in, the staff asked them to verify their phone numbers and quickly eliminated the data quality issue.
Step 4: Implement data cleansing and standardization techniques
When users enter data in different forms, they make mistakes, especially spelling mistakes. They may write “roda” for “road” and forget about it. But when you pick up these values for analysis, they can seriously affect the data set quality. Whenever possible, use a defined list of values or option sets for such fields so that the users cannot make any mistakes. In other cases, normalization tools and techniques can resolve the data inconsistencies to improve the quality of data.
Step 5: Leverage data quality tools and technologies
An enterprise data quality solution like Collibra Data Quality & Observability offers many benefits to organization who need to catch bad data before it causes damage, including Improved data accuracy: Identify and correct errors, inconsistencies, and other issues that can affect data accuracy. Increased productivity: Reduce the amount of manual effort required to maintain data quality, freeing up time and resources for other activities. Enhanced data governance: Provide a centralized platform for managing data governance activities, including data policies, rules, and procedures. Better decision-making: Improve the quality of decision-making based on data. Increased regulatory compliance: Ensure that data is compliant with regulatory requirements, such as GDPR and HIPAA. Improved customer satisfaction: Ensure that customer data is accurate and up-to-date. Cost savings: Reduce the amount of manual effort required to maintain data quality and prevent costly errors, and reduce costs . An enterprise data quality solution like Collibra can help improve the accuracy and reliability of your data, increase efficiency, and support better decision-making.
Step 6: Establish a data-driven culture within the organization Organization-
wide data-driven culture follows a specific set of values, behaviors, and norms that enable the effective use of data. Naturally, it needs a buy-in from everyone to acknowledge their role in data quality. Develop an organization-wide shared definition of data quality, identify your specific quality metrics, ensure continuous measurement on the defined metrics, and plan for error resolutions. Your organization can also leverage Data Governance to standardize the management of data assets and improve their quality. A key recommendation from Gartner is to give business users the ability to flag and address quality problems. With self-service Data Quality, you can further empower data analysts, data scientists, and business users to identify and resolve the quality issues themselves. In short, a robust data-driven culture encourages everyone to contribute to data quality.
Step 7: Appoint data stewards and foster collaboration
As part of the data-driven culture initiative, you can nominate a data steward to manage data quality. Data stewards can analyze the current state of data quality, optimize review processes, and implement the required tools. Overseeing data governance and managing metadata are also part of their responsibility. Having a data steward in the organization ensures clear accountability and complete supervision for improving data quality.
Step 8: Adopt dataOps to empower your teams
DataOps methodology is focused on process-oriented automation along with best practices, to improve the quality and agility of data analytics. Leveraging DataOps can activate data for business value across all technology tiers, from infrastructure to experience. You can innovate with DataOps to add automation to human behaviors that define data quality, test data quality, and remediate data quality failures. Empowering all your teams with the DataOps culture is a strategic way to improve data quality.
Step 9: Implement continuous training and education programs
A data-driven culture ensures participation from the entire organization towards data quality. But it is also essential to sustain their interest and contribution through innovative ideas. Regular training in concepts, metrics, and tool usage will help reinforce the needs and benefits of data quality. Organization-wide sharing of quality issues and success stories can act as friendly reminders. Offering specialized training to staff is an effective approach to improving data quality. Data quality is not just about correcting current errors but also about preventing future errors. Assessing and addressing the root causes of data quality issues in your organization is the key here. Are the processes manual or automated? Are the measurement metrics correctly defined? Can the stakeholders directly correct the errors? Are the data quality techniques correctly incorporated? Is the data quality culture firmly in place? Your data quality strategy should enable the integration of data quality techniques in enterprise applications and business processes for generating higher value from data assets. The data quality solution you choose should focus on delivering continuous data quality across the organization.
Step 10: Monitor, measure, and communicate data quality results
Onboarding everyone in data quality initiatives is critical because data quality today is not limited to a few teams. Making all stakeholders aware of the activities creates interest and promotes participation. If you frequently communicate about data quality errors, possible reasons, initiatives, tests, and results, more people will actively engage with the improvement projects. Documenting the progress, actions, and results further adds to the organizational knowledge base for powering future initiatives.
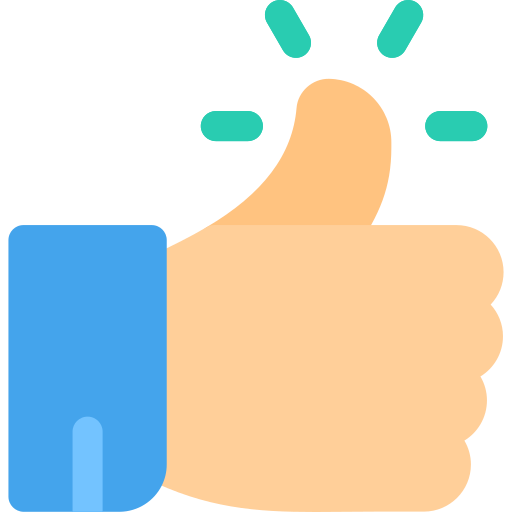
- Managerial Effectiveness!
- Future and Predictions
- Motivatinal / Inspiring
- Other
- Entrepreneurship
- Mentoring & Guidance
- Marketing
- Networking
- HR & Recruiting
- Literature
- Shopping
- Career Management & Advancement